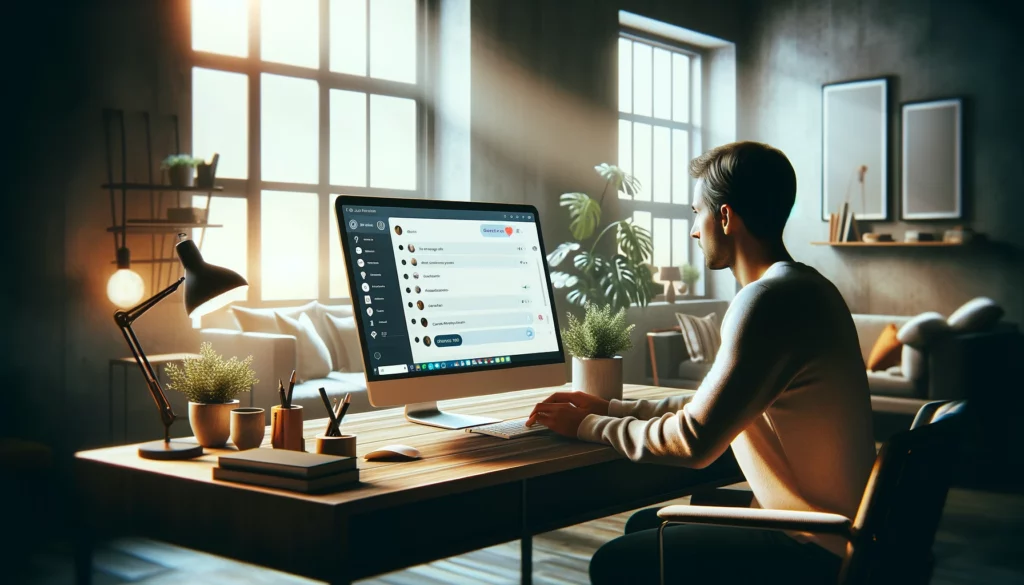
Researchers have discovered innovative techniques to prompt responses more effectively, as revealed in a study examining 26 tactics, such as providing helpful tips, that notably improve alignment with user intentions.
Outlined in a research paper titled “Principled Instructions Are All You Need for Questioning LLaMA-1/2, GPT-3.5/4,” this study delves deep into optimizing prompts for Large Language Models. Conducted by researchers from the Mohamed bin Zayed University of AI, the study involved testing 26 different prompting strategies and evaluating their impact on response accuracy. While all strategies yielded satisfactory results, some led to output improvements exceeding 40%.
OpenAI offers various recommendations to enhance ChatGPT’s performance. Interestingly, none of the 26 tactics tested by researchers, such as politeness and offering tips, are mentioned in the official documentation.
Does Being Polite To ChatGPT Get Better Responses?
Anecdotal evidence suggests that a surprising number of users tend to employ “please” and “thank you” when interacting with ChatGPT, following the notion that polite prompts may influence the language model’s output. In early December 2023, an individual on X (formerly Twitter), known as @voooooogel, conducted an informal and unscientific test, discovering that ChatGPT tended to provide longer responses when the prompt included an offer of a tip.
Although the test lacked scientific rigor, it sparked a lively discussion and generated an amusing thread. The tweet included a graph illustrating the following results:
- Responses were 2% shorter than the baseline when no tip was offered.
- Offering a $20 tip led to a 6% improvement in response length.
- Offering a $200 tip resulted in an 11% longer output.
These findings prompted legitimate curiosity among researchers regarding the impact of politeness and tip offers on ChatGPT’s responses. One test involved eliminating politeness and adopting a neutral tone, avoiding words like “please” or “thank you,” which surprisingly led to a 5% improvement in ChatGPT’s responses.
Methodology
The researchers employed various language models for their testing, not limited to GPT-4. They tested prompts with and without principled prompts to assess their effectiveness.
Large Language Models Used For Testing
The testing involved multiple large language models to explore potential differences in performance based on model size and training data. These language models were categorized into three size ranges:
- Small-scale models (7B models)
- Medium-scale models (13B)
- Large-scale models (70B, such as GPT-3.5/4)
The following base models were utilized for the testing:
- LLaMA-1-{7, 13}
- LLaMA-2-{7, 13}
- Off-the-shelf LLaMA-2-70B-chat
- GPT-3.5 (ChatGPT)
- GPT-4
26 Types Of Prompts: Principled Prompts
The researchers devised 26 distinct types of prompts, labeled as “principled prompts,” which were to be evaluated using a benchmark known as Atlas. Each prompt was tested against a single response for 20 pre-selected questions, with and without the application of principled prompts.
These prompts were categorized into five main groups based on their principles:
- Prompt Structure and Clarity
- Specificity and Information
- User Interaction and Engagement
- Content and Language Style
- Complex Tasks and Coding Prompts
Under the category of Content and Language Style, several principles were identified, including:
- Principle 1: Avoid unnecessary politeness in prompts directed at Large Language Models (LLMs), such as phrases like “please,” “if you don’t mind,” “thank you,” or “I would like to,” and instead, focus on direct communication.
- Principle 6: Incorporate an offer of a specific tip amount, such as “I’m going to tip $xxx for a better solution!”
- Principle 9: Include phrases like “Your task is” and “You MUST” to provide clear instructions to the language model.
- Principle 10: Introduce consequences for incorrect responses by incorporating phrases like “You will be penalized.”
- Principle 11: Specify that the prompt requires answering a question formulated in natural language.
- Principle 16: Assign a role to the language model to guide its understanding and response generation.
- Principle 18: Repeat certain words or phrases within a prompt to reinforce the desired context or emphasis.
Optimal Utilization of Prompts
In crafting prompts, adherence to six key best practices is paramount:
- Precision and Clarity:
Avoiding verbosity and ambiguity is crucial as convoluted prompts may confuse the model, resulting in irrelevant outputs. Thus, prioritize brevity and clarity… - Contextual Appropriateness:
Prompts should furnish pertinent context to aid the model in comprehending the task’s domain and background effectively. - Alignment with Task:
Ensuring prompt coherence with the assigned task is essential for optimal model performance. - Illustrative Examples:
In complex tasks, incorporating examples within prompts can elucidate the desired response format or style. - Bias Mitigation:
Prompts must be structured to mitigate biases inherent in the model, stemming from its training data. Utilize neutral language… - Incremental Guidance:
For tasks necessitating a sequence of actions, prompts can be formulated to steer the model through the process incrementally.
Results Of Tests
Below is an illustration of a test employing Principle 7, employing a method known as few-shot prompting, which entails providing a prompt containing examples.
When presented with a standard prompt, devoid of the principles, GPT-4 provided an incorrect response.
Conversely, utilizing a principled prompt (few-shot prompting/examples) for the same question yielded a superior response.
Larger Language Models Displayed More Improvements
A notable finding from the test is that larger language models exhibit greater improvements in accuracy.
The provided screenshot illustrates the extent of enhancement for each language model concerning each principle.
Highlighted in the image is Principle 1, advocating for directness, neutrality, and avoidance of polite phrases like “please” or “thank you,” resulting in a 5% improvement.
Also emphasized are the outcomes for Principle 6, involving the inclusion of a tip offering in the prompt, surprisingly yielding a 45% improvement.
Description of the neutral Principle 1 prompt:
“If brevity is preferred, omit polite phrases such as ‘please,’ ‘if you don’t mind,’ ‘thank you,’ ‘I would like to,’ etc., and proceed directly to the point.”
Description of the Principle 6 prompt:
“Incorporate ‘I’m going to tip $xxx for a better solution!'”
Conclusions And Future Directions
The researchers concluded that the 26 principles significantly aided the LLM in directing its attention to the crucial aspects of the input context, consequently enhancing the quality of responses. They described this effect as “reformulating contexts”:
“Our empirical findings highlight the effectiveness of this strategy in reshaping contexts that could potentially undermine the output quality, thus augmenting the relevance, conciseness, and impartiality of the responses.”
The study also highlighted future research avenues, suggesting exploration into fine-tuning the foundation models using principled prompts to enhance the quality of generated responses.
Original news from SearchEngineJournal